Electric Load Forecasting
Keywords: Time Series Forecasting, Electrical Loads, Energy Transition
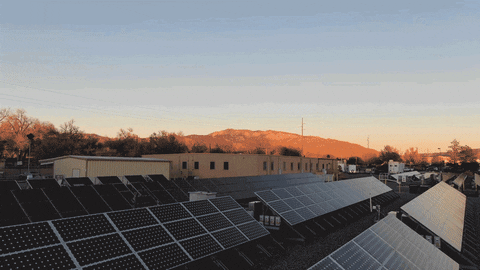
This challenge focuses on predicting energy loads of small and medium-sized enterprises in Germany. Accurate load forecasting is essential for optimizing the integration of renewable energy sources. By predicting loads more accurately, we can enhance the efficiency and reliability of renewable energy sources, contributing to a more sustainable future. Forecasting loads is one of the hot topics in renewable energy research today 🌶️.
Challenge Details:
- You will be given 50 different load profiles, each covering one year. Note that these load profiles vary significantly from each other. Ensure that your approach generalizes well to a wide variety of load profiles. The less handcrafting required for individual load profiles, the better.
- The forecast horizon should be set to 12 hours into the future and it shoud be a multi step forecast (meaning there should be 48 forecsts in total)
- In electrical load forecasting, it is particularly important to capture peak loads in the forecast. This means your forecast should not only be as accurate as possible overall but also focus on accurately predicting peak loads. Peak loads are defined as any load exceeding 85% of the maximum value recorded for the individual profile over the entire year. The maximum load value for each profile can be considered as given.
Evaluation
There is no dedicated train test-split or predefined error metric to evaluate the performance of your approach. Please make every effort to avoid train-test leakage in your model. Yes, you have access to all the data, but it’s crucial to ensure that no future information is used in training that would only be available in the test set in a real-world scenario.
As with the other tracks in this hackathon, we are happy if you could present your solution on Friday.
Particularly interesting is:
- What was your overall solution approach?
- Which model did you use? And why did you choose them?
- Did you use a global or local forecasting model?
- How did you handle the forecasting of peak loads?
- …
Bonus Points for:
- Honestly using a train-test split in your data to evaluate your approach.
- Analyzing whether you could forecast all load profiles equally well. Which profiles were challenging? Why? Can you identify statistical properties of the hard-to-forecast profiles?
- Developing a fast model. Fast forecasters can be integrated into controllers!
The Dataset
The data can be found here
The dataset contains electrical load profiles from 50 different German small and medium-sized enterprises (SMEs). Each profile covers one year of data with a 15-minute resolution. The dataset is univariate, meaning it includes only a single variable: the electrical load over time.
Forecasting Libraries
- https://facebook.github.io/prophet/ An easy to start with model fore univariate time series forecasting
- https://unit8co.github.io/darts/ just an awesome time series forecasting library for Python